As artificial intelligence (AI) rapidly advances, understanding the differences between machine learning (ML) and deep learning (DL) becomes crucial. While often used interchangeably, these two AI subfields have distinct approaches, architectures, and applications. In this blog, we’ll explore the unique features of machine learning vs deep learning, analyze their real-world applications, and discuss how they are impacting various industries.
1. What is Machine Learning?
Machine learning is a branch of AI focused on creating systems that can learn and make decisions from data without explicit programming.
- Data-Driven Learning: ML models learn from structured data to make predictions, classify data, and find patterns.
- Supervised and Unsupervised Learning: ML includes supervised (labeled data) and unsupervised (unlabeled data) learning methods.
- Algorithms: ML relies on algorithms like decision trees, support vector machines, and clustering.
2. Understanding Deep Learning
Deep learning, a subset of machine learning, mimics the structure of the human brain using artificial neural networks.
- Neural Networks: DL leverages complex architectures of layered networks to analyze data at multiple levels.
- Feature Extraction: Unlike ML, DL doesn’t require manual feature extraction; the model learns to recognize patterns autonomously.
- Large Data Sets: DL is especially effective with large datasets and is computationally intensive.
3. Machine Learning vs Deep Learning: Key Differences
While ML and DL both fall under the umbrella of AI, they differ in terms of complexity, data requirements, and application scope.
- Data Requirements: ML performs well with structured, labeled data, while DL thrives with large, complex datasets.
- Computation Power: DL often requires GPUs due to its extensive calculations, while ML can run on less powerful systems.
- Feature Engineering: In ML, feature engineering is often necessary, whereas DL learns features directly from data.
4. Applications of Machine Learning
Machine learning has a wide range of applications across industries, making it a versatile AI approach.
- Finance: ML algorithms detect fraudulent transactions and automate financial trading.
- Healthcare: ML assists in diagnosing diseases through predictive modeling.
- Retail: ML enhances personalized recommendations, inventory management, and customer service automation.
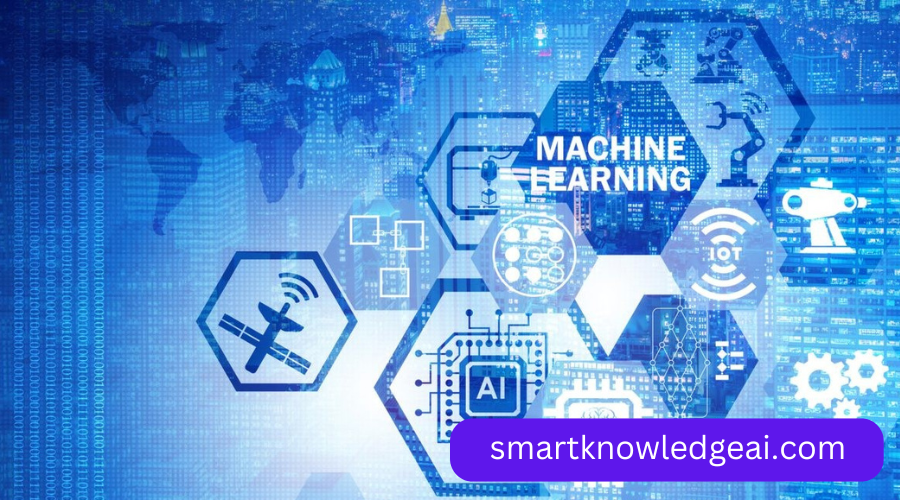
5. Applications of Deep Learning
Deep learning’s ability to handle massive datasets and complex tasks opens new possibilities in cutting-edge technology.
- Image Recognition: DL powers facial recognition and medical imaging analysis.
- Natural Language Processing (NLP): DL underpins NLP applications like language translation and sentiment analysis.
- Autonomous Vehicles: DL is critical in processing sensory data for self-driving cars, allowing for real-time decision-making.
6. Machine Learning in Real Life: Enhancing Everyday Tasks
Machine learning technology is embedded in many daily technologies, from search engines to spam filters.
- Email Filtering: Spam filters use ML to classify and sort emails accurately.
- Recommendation Systems: Platforms like Netflix and Amazon use ML to personalize user recommendations.
- Smart Home Devices: Virtual assistants such as Alexa and Google Assistant use ML for voice recognition and automation.
7. Deep Learning in Real Life: Powering Advanced AI Solutions
Deep learning drives many advanced AI applications that require high accuracy and real-time data processing.
- Voice Assistants: Deep learning enables voice-to-text applications and speech synthesis in devices like Siri.
- Medical Imaging: DL algorithms assist in diagnosing diseases by analyzing radiology images and identifying anomalies.
- Game AI: DL is used to create responsive, intelligent characters in video games, making for immersive experiences.
8. Challenges of Machine Learning vs Deep Learning
Despite their potential, both ML and DL face unique challenges that need to be addressed for widespread adoption.
- Data Quality: Poor data quality affects both ML and DL model accuracy, making data cleaning essential.
- Bias in Models: Bias in training data can lead to biased ML and DL models, potentially causing ethical concerns.
- Computational Requirements: DL, in particular, demands high computational power, making it costly to implement.
9. Future of Machine Learning and Deep Learning
As AI research continues, the future of ML and DL holds exciting advancements in technology and new applications.
- Edge AI: Machine learning on edge devices will enable real-time processing in IoT applications without relying on cloud services.
- Hybrid Models: Combining ML and DL techniques can improve accuracy and efficiency in various applications.
- Enhanced Interpretability: Future ML and DL models aim to provide more transparency, helping users understand decision-making processes.
Conclusion
Machine learning and deep learning are powerful tools within AI, each with distinct applications and benefits. From automated diagnostics in healthcare to recommendation engines in retail, both ML and DL continue to drive innovation across sectors. As technology progresses, the distinctions between these approaches may blur, but understanding their differences remains essential for harnessing their full potential. Embracing both ML and DL technologies promises a future of smarter, more intuitive systems that can transform our world in unprecedented ways.