Introduction
As artificial intelligence (AI) advances and integrates into more areas of daily life, its ethical implications grow in significance. From privacy issues to algorithmic bias, AI has the potential to raise challenging ethical questions that impact society on a global scale. Understanding these challenges is crucial for ensuring AI is developed responsibly. This blog explores the key ethical concerns surrounding AI, including privacy, accountability, and the prevention of bias, and discusses ways to approach ethics of AI development.
1. Privacy Concerns in AI
AI systems often rely on vast amounts of personal data, raising concerns about individual privacy.
- Data Collection and Storage: AI algorithms require data to learn and improve, but collecting this data, especially personal information, raises questions about user consent and data protection.
- Data Anonymity: Despite efforts to anonymize data, AI can sometimes re-identify individuals from anonymized data, threatening user privacy.
- User Consent: In many cases, individuals are unaware of how their data is used by AI systems. Ensuring transparency and obtaining informed consent are essential for ethical AI use.
2. Algorithmic Bias and Fairness
AI algorithms are often trained on historical data, which can contain biases that reflect past social inequalities.
- Bias in Training Data: If the data used to train an AI system reflects historical biases, the AI may reproduce or even amplify these biases in its decisions.
- Fair Representation: AI models must be trained with diverse data to ensure they don’t unfairly disadvantage certain groups or perpetuate stereotypes.
- Algorithm Audits: Regular audits of AI systems help detect and address bias. Implementing checks for fairness is an essential practice for responsible AI development.
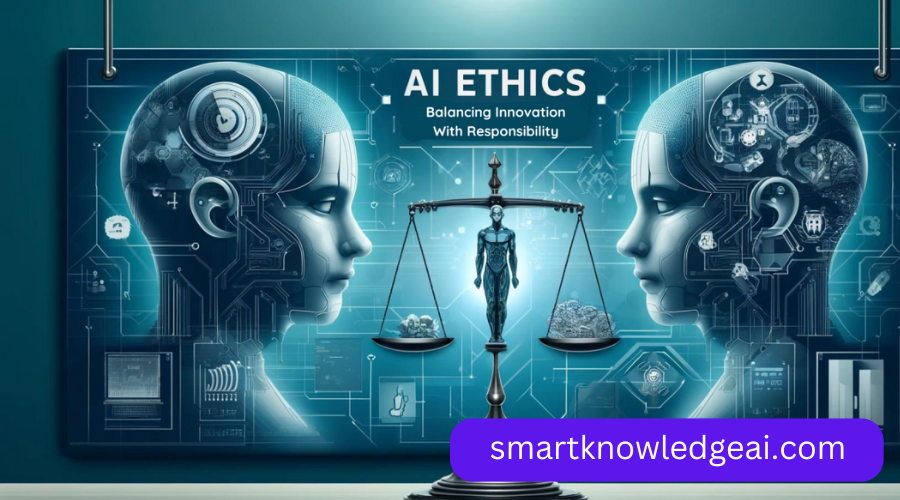
3. Accountability and Transparency
Determining responsibility when AI systems fail or make mistakes is an ongoing ethical challenge.
- Black Box Problem: Many AI models, especially deep learning algorithms, are complex and lack transparency, making it hard to understand how they reach their decisions.
- Clear Accountability: Determining who is accountable when AI causes harm or makes an incorrect decision is essential. Is it the developer, the user, or the system itself?
- Explainable AI: Explainable AI (XAI) is an approach focused on making AI models more transparent, helping users understand the reasoning behind AI decisions.
4. Autonomous Decision-Making and AI Control
As AI systems become more autonomous, questions arise about the extent to which they should be allowed to make decisions without human oversight.
- Limits of Autonomy: While autonomous AI has many benefits, there is a need for guidelines on the types of decisions AI should be allowed to make independently.
- Human-in-the-Loop: Ensuring a human remains involved in critical decision-making processes can prevent unintended outcomes and allows for ethical oversight.
- Regulation and Oversight: Governments and organizations need to establish regulations that control the extent of autonomy AI systems can possess, especially in fields like healthcare and finance.
5. AI and Employment: Ethical Implications of Automation
AI-driven automation impacts the job market, raising concerns about job displacement and the future of work.
- Job Displacement: As AI automates repetitive tasks, there is concern about job loss in certain sectors, affecting low-skilled and vulnerable workers the most.
- Reskilling and Upskilling: To address AI-induced job displacement, it’s crucial to invest in reskilling and upskilling programs, preparing the workforce for new roles that require human skills.
- Social Responsibility: Companies implementing AI should consider the broader societal impact, balancing automation with opportunities for affected employees.
6. Security Risks in AI
AI systems can be vulnerable to security threats, including hacking, data breaches, and adversarial attacks.
- Adversarial Attacks: In adversarial attacks, AI models are exposed to data designed to mislead them, resulting in incorrect outputs. Ensuring AI resilience to such attacks is essential for security.
- Data Breaches: AI systems handling sensitive data must be safeguarded against unauthorized access, as breaches can have severe implications for individuals’ privacy.
- Ethical Hacking: Companies can use ethical hacking to test AI systems’ defenses, helping to identify and patch vulnerabilities before malicious actors can exploit them.
7. Environmental Impact of AI
The development and training of AI models, particularly large ones, require significant energy, raising ethical concerns about environmental sustainability.
- Energy Consumption: Training large AI models consumes a considerable amount of electricity, often sourced from non-renewable energy, which increases carbon emissions.
- Sustainable AI Practices: Companies can adopt sustainable AI practices, like using renewable energy sources and optimizing models to be more energy-efficient.
- Responsible Model Training: Reducing the need for retraining and reusing pre-trained models can help reduce AI’s environmental footprint, promoting more sustainable technology development.

8. The Role of Regulations in Ethical AI Development
Governments and institutions play an essential role in setting guidelines and regulations to ensure ethical AI practices.
- AI Ethics Policies: Countries are establishing ethics of AI policies that mandate responsible AI development and limit harmful practices. These policies set industry-wide standards and hold organizations accountable.
- Transparency Requirements: Regulations around transparency ensure that users are informed about AI’s role in decision-making, promoting trust and accountability.
- Global Collaboration: Ethical AI requires a collaborative global approach, where countries work together to establish standards and ensure ethical practices across borders.
9. Balancing Innovation and Ethics
Striking a balance between advancing AI technology and maintaining ethical standards is a key consideration for developers.
- Encouraging Responsible Innovation: Developers should prioritize ethical considerations as they innovate, ensuring their solutions benefit society without causing harm.
- Ethical AI Research: Funding and support for ethical AI research encourage innovation while adhering to moral standards, helping develop AI solutions that consider the broader social impact.
- Long-Term Impact Assessment: Considering the long-term societal impact of AI can help guide ethical decisions and promote sustainable progress in AI technology.
10. Promoting AI Ethics Through Education
Educating developers, policymakers, and the public about AI ethics can help create a more informed society that advocates for responsible AI practices.
- Ethics in AI Curriculum: Incorporating AI ethics into educational programs ensures that future developers understand the importance of responsible AI development.
- Public Awareness: Educating the public about AI’s ethical implications allows individuals to make informed choices about the technologies they use and advocate for ethical AI standards.
- Ethical Guidelines for Companies: By adopting ethical guidelines, companies can create a culture of responsibility, ensuring that all team members consider the societal implications of their AI projects.
Conclusion
AI has the potential to transform industries and improve lives, but these advancements come with ethical considerations that cannot be ignored. From data privacy to environmental sustainability, the challenges are complex, requiring a commitment to responsible development practices. By addressing these issues and fostering a culture of ethical AI, we can create technology that enhances society without compromising values. Embracing ethical AI principles will pave the way for a future where technology and humanity coexist harmoniously, ensuring a positive impact on future generations.